Project Summary
Inertial Sensing Based on
Quantum-Enhanced
Levitation Systems
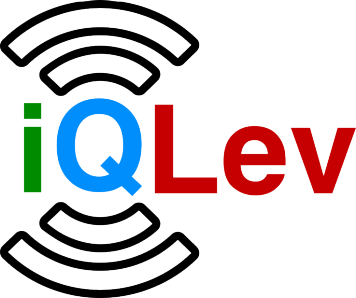
Information and data are becoming increasingly important both to European society and to the European economy. It will be key to the future of Europe to start having an appropriate share in the development and control of central information and data related sectors. One of these central sectors - the Internet of Things - is currently being developed world-wide, has a compound annual growth rate between 10 and 30%, and is starting to influence our daily lives.
Sensors are a fundamental building block of the Internet of Things. For instance, cars would be boosted in the direction of fully self-driving vehicles, if an inertial sensing system could precisely measure their position at every instant of time. Within the framework of the Internet of Things, globally connected self-driving cars could contribute to less congestion, less pollution and an overall improvement in quality of life.
It is the aim of iQLev to join in shaping such a positive European vision by developing high-end inertial sensing systems for navigation, positioning and gravimetry/seismometry. Our revolutionary approach combines quantum-enhanced sensing with noise cancellation via perfect system isolation through levitation. This combination results in inertial sensing performances beyond currently possible sensitivities. Optical, electrostatic and magnetic levitation are investigated via an effective, parallel and synergetic approach. The capabilities of our inertial sensors for linear acceleration and rotation (gyroscope) sensing in the low frequency domain (mHz) are evaluated and benchmarked according to industry standards. The first target areas of the sensors under development are scientific satellite missions and seismic measurements.
iQLev is a external pageFETOPENcall_made project within the RIA - Research and Innovation actions. The external pageprojectcall_made started on 1st of January 2020 and has a duration of three years.
Funding
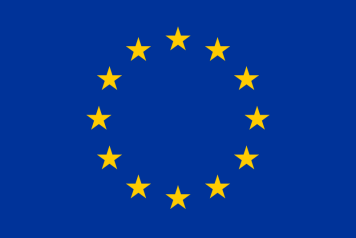
This project has received funding from the European Union’s Horizon 2020 research and innovation programme under grant agreement No 863132.